HARNESS THE POWER OF YOUR CUSTOMER DATA
NGDATA's AI-powered Intelligent Engagement Platform creates meaningful digital experiences for your customers
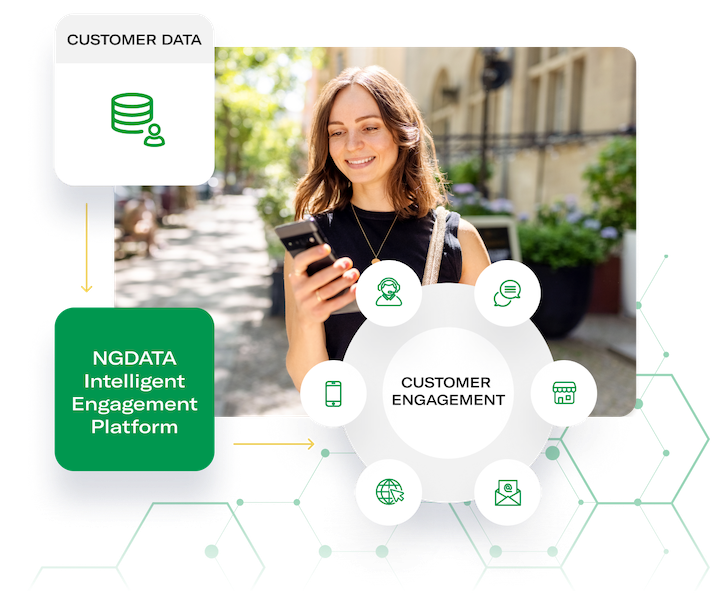
DO NOT ADD CONTENT ABOVE HERE
Discover the most relevant audiences and moments to engage
Acquire, onboard, and cross-sell to create loyal customers
10+ years powering half-a-billion data-driven experiences
Expert onboarding, implementation, and optimization services
DO NOT ADD CONTENT BELOW HERE